Art Restoration Using AI: A Renaissance of Lost 17th-Century Titian Paintings
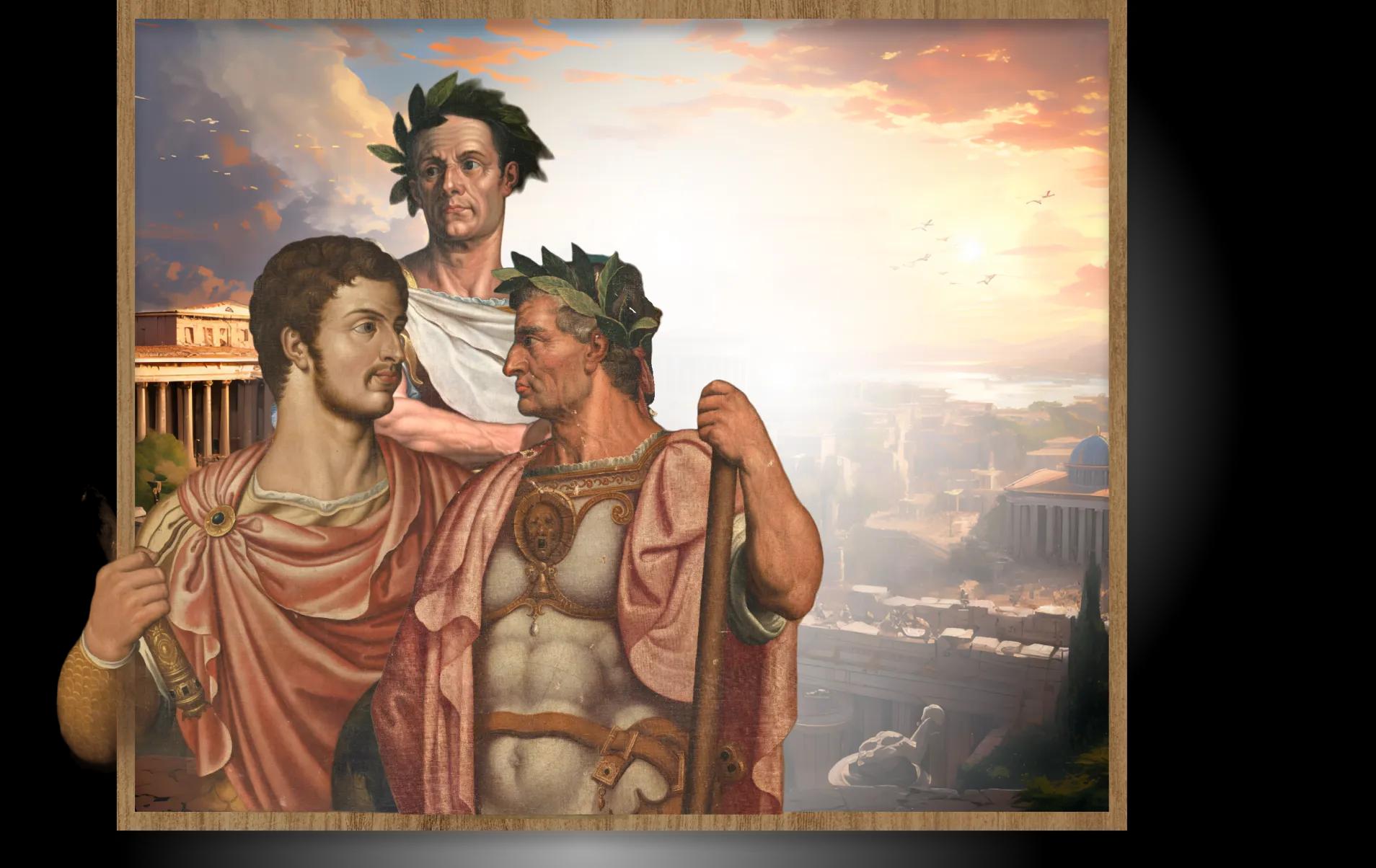
Client Profile
Client profile
Objective
The primary objective was to recreate lost Titian paintings by leveraging historical sketches, engravings, and preserved artworks, ensuring the result stayed true to the unique style and artistic quality of 17th-century masterpieces.
An additionall key goal was to develop an AI model capable of generating high-quality images that accurately reflect the aesthetics and techniques of historical periods, thus creating a tool that can serve both artistic preservation and future collaborations in the art restoration domain.
Additionally, we developed a series of NFTs dedicated to the restored works. This initiative aimed to modernize the approach to art preservation while opening new avenues for audience engagement and project monetization.
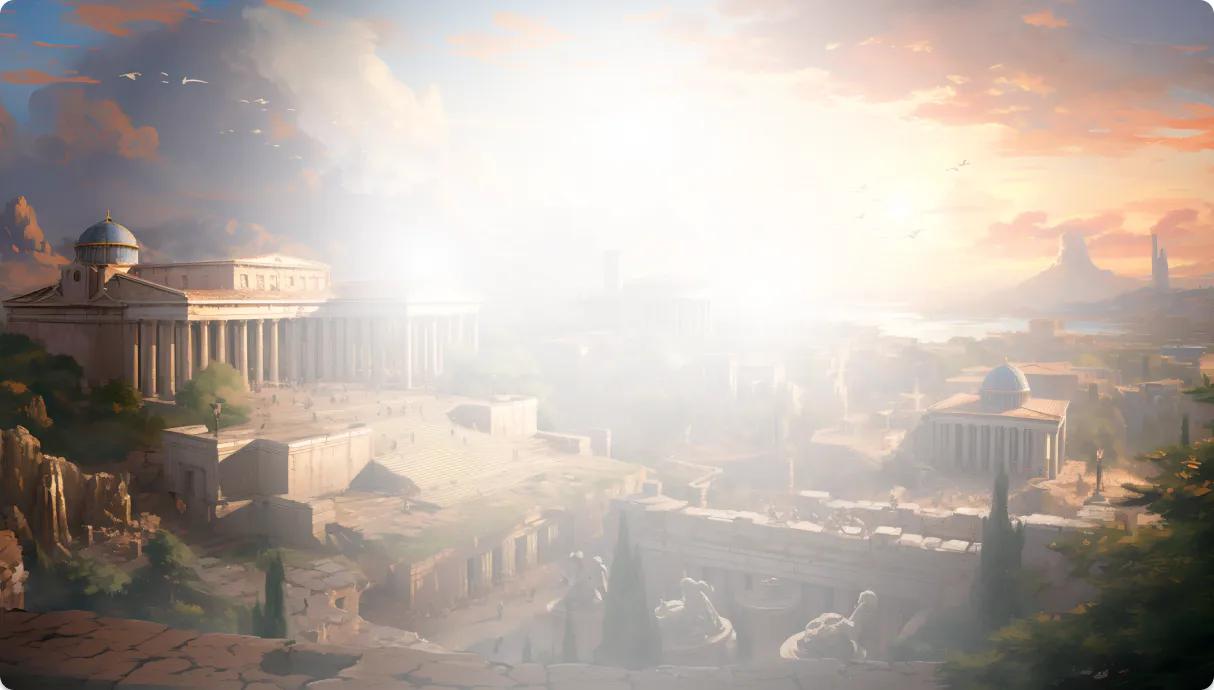
Challenge
Capture the nuances of Titan's Brushwork and Technique
Historical color accuracy
Historical accuracy in elements and composition
Team Composition
- 1
Project Manager
- 1
Design Lead
- 2
ML Developers
- 2
Web Developers
7
months of engagement
Business Analysis & Information Gathering
When the art experts approached Monosoft, they had only an idea about recreating the lost Titian paintings. Through close collaboration with the client, including professor Hassam from London, and detailed analysis of preserved Titian works, we collected the necessary data to build our models. We focused on iterative experimentation, evaluating over 50 different models to refine the process, including developing unique datasets based on Renaissance artists like Titian. Our approach also involved hiring a designer to create precise Caesar's outlines, which went through several review iterations. We developed an in-depth product requirements document (PRD) to ensure the final output met the clients' vision.
Solution
Monosoft used Stable Diffusion (open source) and LoRA to train the AI model. We continuously experimented with various training techniques, trying more than 50 model variations to achieve the best quality images. By integrating LoRA with a main model consisting of 7700 images (SDXL and Flux), the LoRA network adjusted parameters in the core model for more accurate results. Through rigorous testing and adjustments, we recreated the lost paintings in a way that closely matched the original 17th-century style. Additionally, we developed a custom platform for generating these images, allowing for future collaborations and improvements. We employed tools to verify the originality of the generated images and used statistically weighted feedback from other networks to enhance the quality of the output.
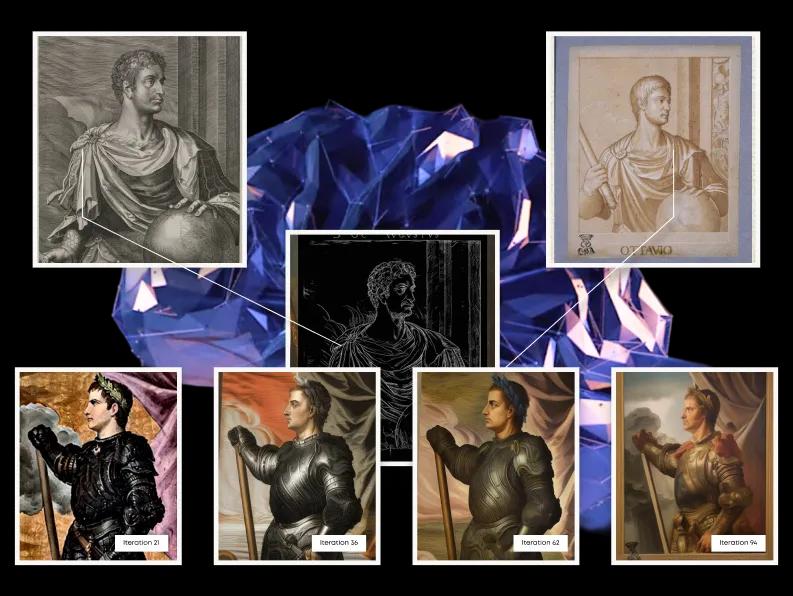
Engagement Timeline
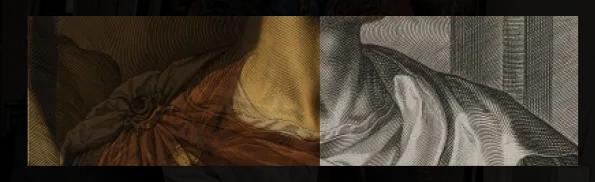
Original Titian's technique
We achieved an almost perfect result of mirroring the original piece’s dimensions and techniques used by Titian.
Paint color pigments
As was confirmed by numerous tests, the color shades used in generated pictures look like they were made the same way as in the old days, copying the result of mixing paints by hand.
Authenticity of Images
The images generated by AI achieved 92% authenticity scored by ResNet neural network in comparison to the original Titian works.
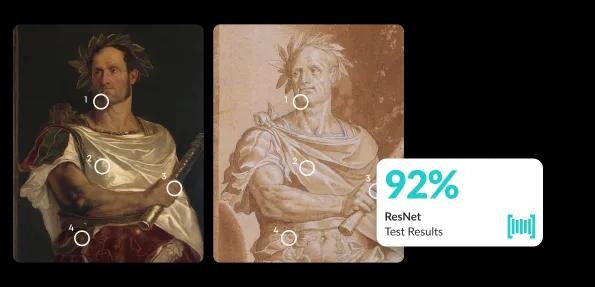
Consistent Generation Results
The AI maintained a stable index of 92%, creating authentical images.
Iterative Testing and Adjustments
Over 50 models were tested, with different techniques applied to various regions of the image for color and texture accuracy.
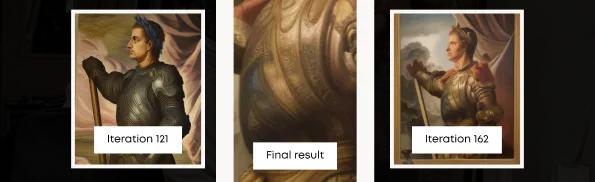
Custom Platform
We developed a unique platform to streamline the generation and refinement process of historical artwork.
Collaboration Across Networks
LoRA worked in conjunction with the main model, ensuring adaptive corrections to fine-tune image details.
Key Features
We achieved an almost perfect result of mirroring the original piece’s dimensions and techniques used by Titian.
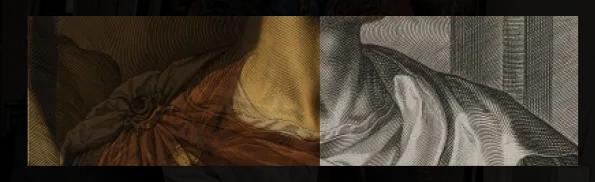
As was confirmed by numerous tests, the color shades used in generated pictures look like they were made the same way as in the old days, copying the result of mixing paints by hand.
The images generated by AI achieved 92% authenticity scored by ResNet neural network in comparison to the original Titian works.
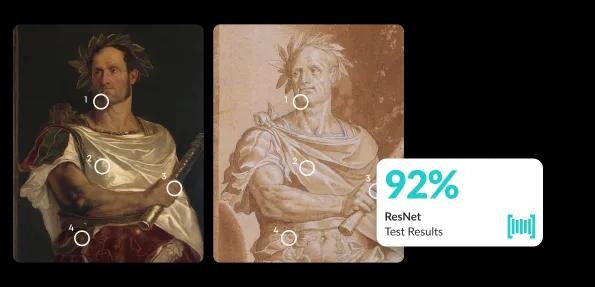
The AI maintained a stable index of 92%, creating authentical images.
Over 50 models were tested, with different techniques applied to various regions of the image for color and texture accuracy.
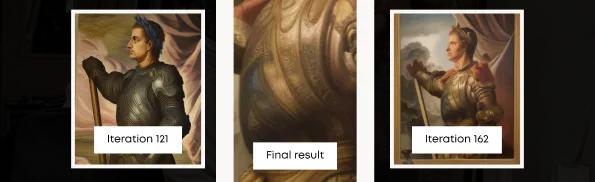
We developed a unique platform to streamline the generation and refinement process of historical artwork.
LoRA worked in conjunction with the main model, ensuring adaptive corrections to fine-tune image details.
Results And Impact
92%
of authenticity scored by ResNet neural network
80%+
test results of authentication images
The generated images closely matched the artistic style and quality of the original 17th-century Titian paintings, creating a powerful restoration tool for art experts.
The project enabled the clients to establish a business model based on the AI-generated images, with opportunities for collaboration with other artists.
The restored paintings were not only marketable but also contributed significantly to the preservation of cultural heritage.
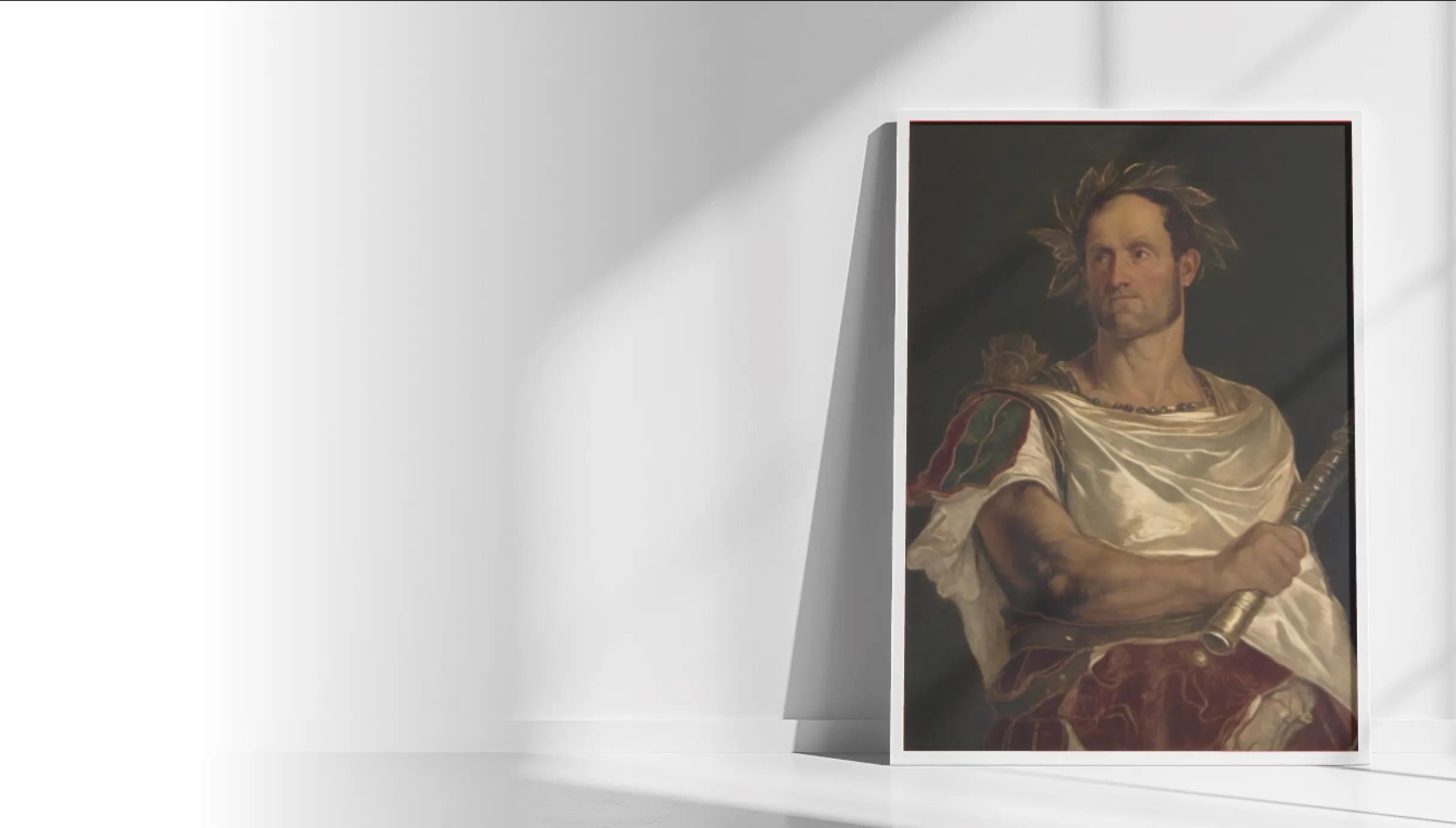